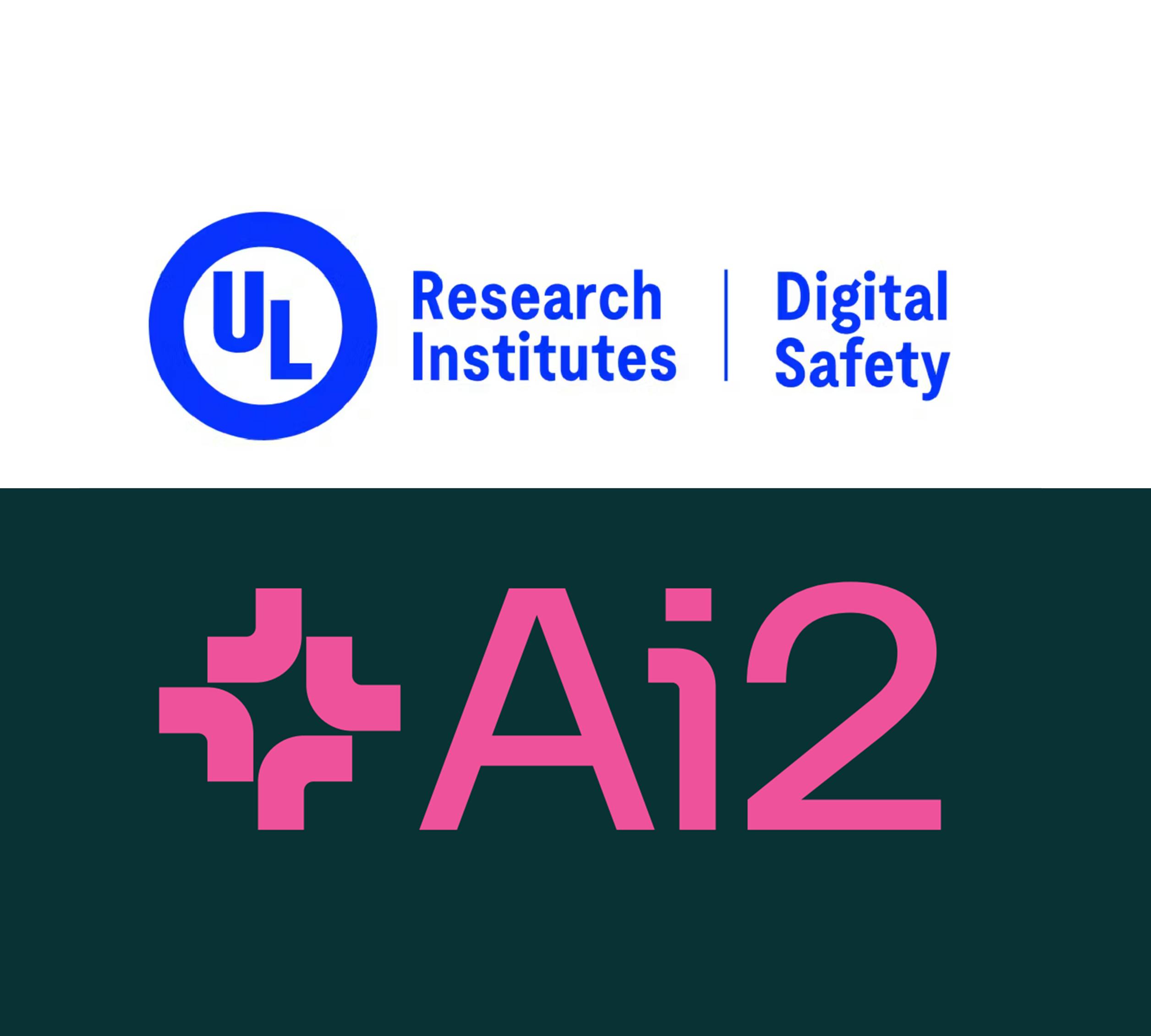
Tabletop Red-Teaming for AI Safety: Leveraging the Hypothetical to Inform the Practical
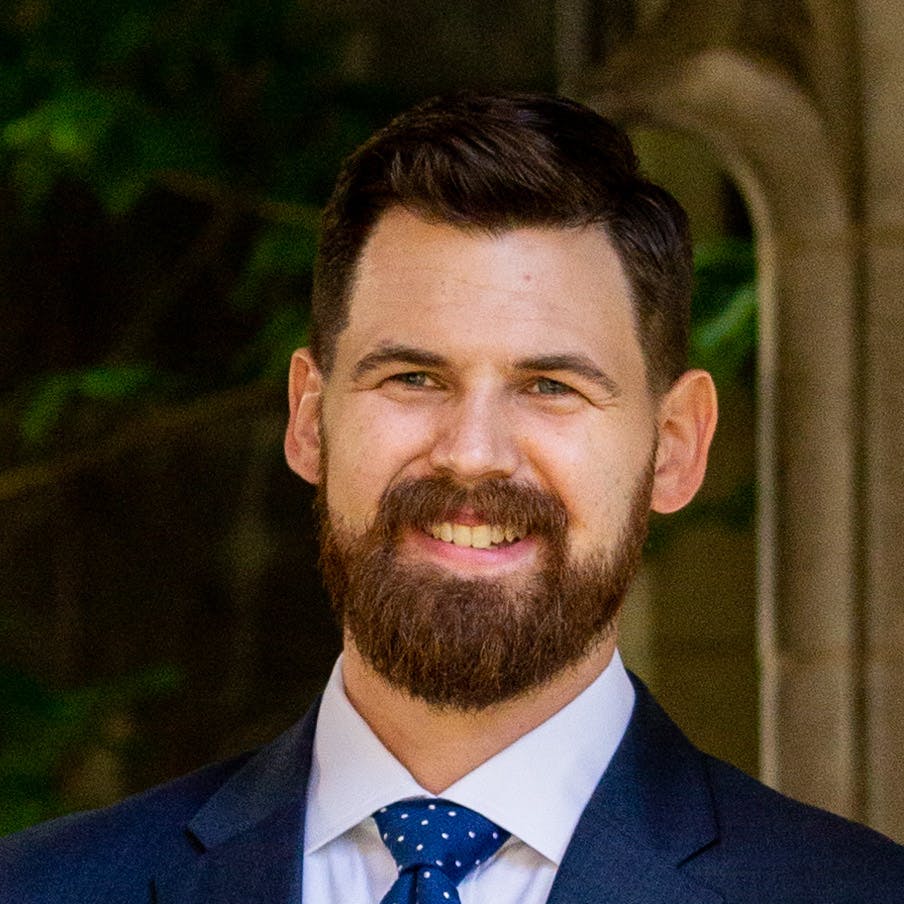
with Will Smith, legal counsel, The Allen Institute for Artificial Intelligence
For many people in the field of artificial intelligence (AI), “red-teaming” means prompt-based adversarial testing. That isn’t the only kind of AI red-team, however. A kind of exercise we call “tabletop red-teaming” — a series of workshops that involve a diverse cross-section of team members from an organization working on an AI project, which concludes in the delivery of a concrete report with a clear action plan — provides an early opportunity to identify a project team’s blind spots, brainstorm opportunities and threats, challenge assumptions, and improve AI deployment plans.
The Digital Safety Research Institute (DSRI) and The Allen Institute for Artificial Intelligence (Ai2) organized tabletop red-teams to assess the opportunities and risks involved in Ai2’s release of the Open Language Model (OLMo) family of large language models (LLMs). We ran these workshops just over a year ago, in November 2023, and designed them by adapting practices that have been used successfully in other software contexts for the particular needs of AI research. We deferred reflecting on these workshops until we could assess the impact that they had on our ongoing, joint work in AI safety.
DSRI and Ai2 can now report that tabletop red-teaming had a positive impact on Ai2’s programs, both immediately and over a longer time horizon, as described in this post.
As an AI safety intervention, tabletop red-teams require relatively few resources — just one or more facilitators, staff time, and the space (or video conferencing software) to support events. Although the exercises themselves are relatively fast, we found their impact can be lasting: With DSRI facilitating, Ai2 conducted a bottom-up re-evaluation of its AI safety posture and presented a concrete action plan to organizational leaders at the director level all in less than three days of active work, paced out over a few weeks. This action plan became the blueprint for Ai2’s safety objectives during the 2024 calendar and served as a continual touchpoint for Ai2’s nonprofit, public interest research and educational activities.
We believe DSRI and Ai2’s experience serves as an instructive example for similarly situated organizations that might otherwise overlook the benefits of structured reflection to inform and support the technical investigation of AI safety. We found that taking tabletop hypotheticals seriously generated meaningful gains in real-world practice. Here’s that story:
Immediate Results. Starting with three workshops facilitated by DSRI in the fall of 2023, Ai2 was able to bring into focus three critical questions for the organization in the pre-release environment:
- What is the organization's definition of “safety”?
- Consideration here included defining the procedures and documentation that the organization would need to determine when an AI artifact has met the organization’s internal criteria for safety.
- Who at the organization owns “safety”?
- Discussion here focused on a range of accountability questions, including which group or project team should have responsibility for articulating safety standards, identifying appropriate benchmarks and assessments, and monitoring artifact or system performance post-release, respectively.
- What is the organization's incident response plan?
- Attention was given here to scenarios in which a released artifact did not meet the organization’s internal standards or exhibited unexpected behaviors. In particular, the organization reflected not only on responding to failure modes, but also on reverse-engineering such cases to identify appropriate mitigations or prior decision points at which the undesired outcome might have been averted.
Long-Horizon Results. Oriented by these critical questions, Ai2 set about addressing them in sequential fashion over the course of the year ahead, starting with developing appropriate processes for identifying and mitigating risks in advance of the public release of the first version of OLMo 7B. The results of Ai2’s longer term work during 2024 were ultimately responsive to each of the safety questions that emerged from the tabletop exercise.
- Meaning of AI Safety: Ai2 defined the meaning and scope of safety at an organizational level, resulting in the publication of Ai2’s principles for approaching AI safety.
- In-House Safety Review Pilot: Ai2 designed and launched a pilot program organized around an in-house safety team that would act as a single point of contact for project teams and facilitate safety reviews for every major release on Ai2’s calendar (as well as other projects at an early stage in the development lifecycle). Among other things, the Ai2 safety team researched and published the first version of Ai2’s responsible use guidelines, which inform both expert and lay users about the scientific research purposes, intended uses, and limitations of Ai2’s artifacts.
- AI Safety Toolkit: To help mitigate known harms that arise from LLMs, Ai2 developed the Ai2 Safety Toolkit, a central hub for advancing LLM safety and fostering open science. The Ai2 Safety Toolkit is a suite of resources focused on LLM Safety that includes: WildTeaming, an automatic red-teaming framework for identifying and reproducing human-devised attacks; WildJailbreak, a high-quality, large-scale safety training dataset for safety fine-tuning; and WildGuard, a light-weight, multi-purpose moderation tool for assessing the safety of user-LLM interaction.
Overall Assessment. We found that DSRI’s tabletop red-teaming exercises helped Ai2 to develop appropriate processes for identifying and mitigating risks in advance of the public release of highly capable AI systems, beginning with OLMo 7B and progressing to Molmo, Tülu3, and OLMo2. The workshops also helped Ai2 and DSRI identify opportunities for research into AI safety in the open and for the benefit of the scientific community that depends on open and transparent AI resources to investigate the effectiveness of concrete, frontline safety interventions. This preparatory tabletop work also built a bridge to traditional adversarial red-team exercises co-organized by DSRI and Ai2 at the second Generative Red Team event at DEFCON AI Village, where we experimented with new means for AI system flaw reporting.
Next Steps. One of the most important outputs from the tabletop exercises for DSRI and Ai2 was the framing of AI safety problems as research problems. AI safety presents a series of specific, empirically verifiable applied problems that research must solve. Importantly, these problems motivate research not only in human-computer interaction and related fields, but also in machine learning and areas of computer science far upstream from real-world deployment of AI systems.
DSRI and Ai2 see opportunities for future work in these areas such as:
- Scalable, effective independent flaw reporting mechanisms;
- Best practices for writing and annotating testing datasets to preserve test integrity;
- Pre-deployment and limited-release testing methods in real-world use cases;
- Measuring the success of efforts to improve training datasets and processes in response to creative and scientific community feedback;
- Reproducibility and transparency in model testing and development.
DSRI and Ai2 can share more details about the process of tabletop red-teaming upon request, including the structure of each workshop session and the materials used to structure and prepare for the workshop. Both organizations are interested in helping other research institutions benefit from our experience with this process. DSRI’s workshop design drew loosely on methods called Open Space Technology and more closely on what the U.S. military once called “the Red Team Handbook,” which is a collection of group exercises drawn from a wide variety of sources in organizational behavior, management, and related fields. As we developed these exercises, we saw practitioners in AI using the Delphi method and other means of expert consultation to help with forecasting and decision-making about uncertain future threats. But we saw fewer reports about how organizations used internal exercises to develop their own understanding. This seemed to us like a missed opportunity.
Previous DSRI blog posts describe some of the automated, repeatable assessments for privacy protection and context-specific assessments for AI advice, we have conducted while evaluating Ai2’s OLMo models. As this post shows, collaborating around the results of AI evaluation is just one way that AI research can benefit from engaging with independent assessment. Tabletop exercises can motivate action at the organizational level that accelerates technical progress across a range of projects. This post gives a quick overview of our experience with this kind of safety planning at the organizational level.